Top 5 challenges faced by Data Scientists and how to overcome them
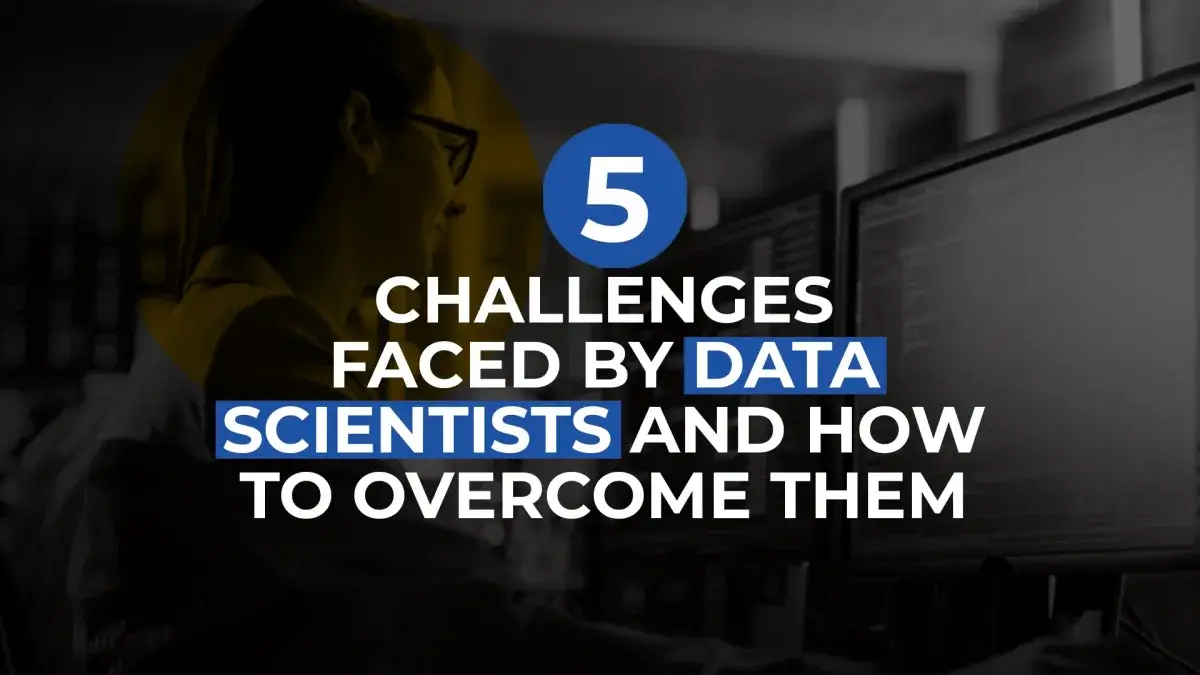
Here's What We've Covered!
- How can AI-enabled data science help data scientists?
- What challenges do data scientists face?
- Final Thoughts
- FAQs
- What are the major issues in data science?
- What are the hardest problems in data science?
- What is the biggest challenges in data science?
- What is the central challenge of data science?
- How does AI-enabled data science help in overcoming data scientist challenges?
- What role does AI play in automating repetitive tasks in data science?
- What strategies can data scientists employ to find and access the right data?
- How can data scientists ensure clean and accurate data for analysis?
- What tools and technologies can data scientists leverage to handle large volumes of data?
- How can data scientists balance data security and accessibility?
Data science has emerged as a critical field in recent years, as companies across various industries seek to harness the power of big data analytics to make informed decisions and drive growth. Data scientists are in high demand, but they often encounter numerous challenges in their day-to-day work. In this article, we will explore the top five challenges faced by data scientists and discuss effective strategies for overcoming them.
But before we dive deep and decode the solutions to major challenges data scientist face, let us discover:
How can AI-enabled data science help data scientists?
Let’s talk about how AI-enabled data science can be a game-changer for data scientists. Buckle up, because this is exciting stuff!
Data scientists have the challenging task of extracting valuable insights from vast amounts of data. That’s where AI comes in like a superhero sidekick, ready to lend a helping hand. With AI-enabled data science, data scientists can supercharge their analytical capabilities and unlock new possibilities.
Automating repetitive tasks
First off, AI can assist in automating repetitive and time-consuming tasks. Think about the hours spent cleaning and preprocessing data—AI algorithms can take care of that. They can identify patterns, anomalies, and outliers in your data, saving you precious time and energy. This means you can focus on the more exciting aspects of your work, like exploring complex algorithms and building innovative models.
Enhancing accuracy of data analysis
But it doesn’t stop there. AI can also enhance the accuracy and efficiency of data analysis. With machine learning algorithms, you can uncover hidden patterns, make more accurate predictions, and gain deeper insights from your data. AI algorithms can learn from existing data, adapt to new information, and continuously improve their performance. It’s like having a super-smart assistant working alongside you, bringing an extra layer of intelligence to your data science projects.
Streamlining workflows
AI-powered tools and platforms are also available to streamline the data science workflow. They provide intuitive interfaces, powerful visualization capabilities, and collaborative features, making it easier for data scientists to work with complex data sets. These tools can accelerate the development and deployment of models, allowing you to iterate and experiment more efficiently.
Simply data analysis at scale
Now, let’s talk about scalability. AI-enabled data science can handle large-scale data with ease. Traditional methods may struggle to process massive volumes of information, but AI algorithms can handle the heavy lifting effortlessly. This opens up exciting opportunities for tackling complex problems and extracting insights from big data that were previously out of reach.
Present volumes of data into bite size information
But wait, there’s more! AI can help data scientists stay ahead of the game by keeping them up to date with the latest research and techniques. AI algorithms can analyze vast amounts of scientific papers, blogs, and articles, extracting key insights and presenting them in a digestible format. This means you can stay in the loop, discover new methodologies, and continuously expand your knowledge and skills.
In a nutshell, AI-enabled data science is a game-changer for data scientists. It automates mundane tasks, improves accuracy and efficiency, streamlines workflows, handles large-scale data, and keeps you informed about the latest developments in the field. So, embrace the power of AI and let it be your trusty ally in your data science adventures. The possibilities are endless, my friend!
Now, let’s go ahead and understand top 5 challenges and their solution to help current and aspiring data scientists improve their performance and achieve higher efficiency to experience faster career growth.
Also Read – Everything You Need to Know About Data Science Jobs
What challenges do data scientists face?
1. Finding and Accessing the Right Data
Challenges in Locating Relevant Data
One of the primary challenges in data science is finding and accessing the right data for analysis. With the exponential growth of data generated by organizations, it can be difficult for data scientists to locate relevant datasets, especially when they are scattered across numerous sources and systems. This challenge is further compounded by the lack of proper data documentation and organization in many companies.
Overcoming Data Discovery Challenges
To address these challenges in big data analytics, organizations should invest in creating a centralized data repository, such as a data warehouse or data lake. This enables data scientists to access all available data assets from a single location, making it easier to find relevant datasets.
Additionally, implementing a data catalog or data dictionary can help improve data discovery. These tools provide metadata and context about each dataset, making it easier for data scientists to understand and locate the information they need. Proper data documentation is crucial for efficient data discovery and should be regularly updated and maintained.
Bonus TIP: If you plan to take up a course in Data Science to make your foundation strong in this sector, make your you enrol with an institution where mentors are experienced professionals, who can pass on their learnings and valuable knowledge to make you job-ready.
Become a Certified Data Scientist from one of India’s Top 5 Analytics Institute – IMS Proschool
2. Data Quality and Cleaning
Challenges in Ensuring Clean and Accurate Data
Data quality is a significant concern for data scientists, as it directly impacts the accuracy and reliability of their analyses. Unfortunately, real-world data is often messy, incomplete, or inconsistent, requiring substantial pre-processing and cleaning before it can be used in analysis. Data cleaning can be a time-consuming and tedious process, taking up a significant portion of a data scientist’s workload.
Overcoming Data Quality Challenges
To tackle data quality challenges in data science, organizations can implement data validation and data cleansing tools. These tools can help automate parts of the data cleaning process, such as identifying and correcting errors, filling in missing values, and removing duplicates. By automating these tasks, data scientists can focus on more complex aspects of data cleaning, ultimately saving time and ensuring high-quality data for analysis.
3. Handling Large Volumes of Data
Challenges in Big Data Analytics
As organizations continue to generate increasingly large volumes of data, data scientists face the challenge of handling and processing these massive datasets. Traditional data processing tools and techniques may not be well-suited for big data analytics, leading to performance issues and longer processing times.
Overcoming Big Data Challenges
To address the challenges in big data analytics, data scientists can leverage advanced tools and technologies designed for processing large volumes of data. These include distributed computing frameworks like Hadoop and Spark, as well as cloud-based data storage and processing platforms. By utilizing these technologies, data scientists can efficiently handle and analyze large datasets, even in real-time.
Bonus Tip: Learning trending tools can make your work much easier! So opt for right course that includes programming languages like Python, R, SAS, Tableau and Tensor Flow and teaches latest ways of using these tools to solve the real life complicated volumes of dat.
Become a Certified Data Scientist in 6 months!
4. Balancing Data Security and Accessibility
Challenges in Data Privacy and Compliance
Data security and compliance are critical concerns for organizations handling sensitive information. As data scientists often require access to confidential data, striking the right balance between data security and accessibility is essential. Ensuring strict adherence to data protection regulations, such as GDPR, while providing data scientists with the necessary access to data can be challenging.
Overcoming Data Security Challenges
To address data security challenges in data science, organizations should implement robust data governance policies and practices. This includes data access controls, data encryption, and data anonymization techniques. Data catalogs can also aid in managing data access by allowing administrators to grant or restrict access to specific datasets based on user roles and permissions, ensuring that data scientists have access only to the data they need while maintaining data security and compliance.
5. Communicating Results to Non-Technical Stakeholders
Challenges in Data Storytelling
Data scientists must effectively communicate their findings and insights to non-technical stakeholders, such as business executives and managers. This can be challenging, as data scientists often have technical backgrounds and may struggle to translate their analyses into clear and actionable business insights. Additionally, poorly defined business terms and KPIs can make it difficult for data scientists to understand and explain the impact of their work.
Overcoming Communication Challenges
To overcome communication challenges in data science, data scientists should practice data storytelling techniques. By presenting their findings in a compelling narrative, supported by visualizations, they can effectively convey the value and impact of their work to non-technical stakeholders. Furthermore, organizations should establish clear definitions for key business terms and KPIs, ensuring that all departments have a shared understanding of these metrics. This will enable data scientists to better align their analyses with business goals and communicate their results more effectively.
Also Read – How Much Do Data Scientists Earn in India, North America, and Europe?
Final Thoughts
Navigating the challenges in data science can be a daunting task for data scientists, but by implementing effective strategies and leveraging the right tools, these obstacles can be overcome. By addressing data discovery, data quality, big data analytics, data security, and communication challenges, organizations can empower their data scientists to unlock the full potential of their data, driving better decision-making and business growth.
If you are interested in learning data science to strengthen your position in the professional world, check out the Data Science Course offered by IMS Proschool that provides you Flexible Learning, Placement Assistance and In-demand certifications. So what are you waiting for, it’s time to upskill yourself and command the true value that you deserve by pursuing this 6 months certification course in Data Science.
FAQs
-
What are the major issues in data science?
Picture this: You are a super-smart detective, but you can’t find the clues to solve the mystery, or the clues are so messy that you can’t make head or tail of them. That’s precisely how data scientists feel when they can’t find or access relevant data or when the data is of poor quality. Now add to it the Everest-like volumes of data they need to handle, while also balancing between data security and accessibility. It’s like trying to walk a tightrope while juggling plates. Then there’s the fun part: explaining all these complex analyses to your boss, who probably thinks Python is just a snake.
-
What are the hardest problems in data science?
One of the hardest problems in data science? Big Data. It’s like being handed a pot of Biryani that’s large enough to feed the entire population of Bangalore and then being asked to find that one piece of elaichi (cardamom) hiding somewhere. Sure, it’s challenging, but that’s where the excitement is, right? Then there’s the issue of data quality. Imagine trying to understand a Hindi movie plot from a poorly subtitled English version. Now, that’s what poor data quality feels like.
-
What is the biggest challenges in data science?
The biggest challenge? Communication, my friend. Data scientists sometimes feel they are speaking Klingon in a room full of non-Trekkies. You can uncover the most groundbreaking insights from your data, but if you can’t explain them to your non-technical boss or stakeholders in a language they understand, well, you’re pretty much up a creek without a paddle. So, yes, brushing up on your storytelling skills might be a good idea.
-
What is the central challenge of data science?
If data science was a Bollywood movie, finding and accessing the right data would be its central plot. Imagine having the recipe to make the perfect Paneer Tikka, but you can’t find the paneer in the vast land of your refrigerator. That’s what data scientists often deal with. And it’s not just about finding the right data, but also ensuring that it’s organized, clean, and usable. Now, wouldn’t that be a blockbuster ending!
-
How does AI-enabled data science help in overcoming data scientist challenges?
AI to data scientists is like an automatic chapati maker to a cook. It simplifies routine tasks, such as cleaning data, identifying patterns, and learning from past decisions. It’s like having a digital intern that never sleeps!
-
What role does AI play in automating repetitive tasks in data science?
AI in data science is like the repeated use of “Kabhi Khushi Kabhie Gham” in a Karan Johar movie. It takes care of the repetitive tasks – cleaning data, filling missing values, and running similar analyses – allowing data scientists to focus on the more creative aspects of their work.
-
What strategies can data scientists employ to find and access the right data?
Finding the right data is a bit like finding your soulmate in a country of a billion people. It requires patience, strategy, and a good sense of direction. Techniques like data mining, web scraping, and usage of APIs can often lead to the “right one.”
-
How can data scientists ensure clean and accurate data for analysis?
Ensuring clean data is like doing your spring cleaning – it requires diligence, attention to detail, and the right tools. Techniques like data cleansing and data validation come in handy here.
-
What tools and technologies can data scientists leverage to handle large volumes of data?
Data scientists use a range of tools like Python, R, SAS, Hadoop, and SQL to handle large volumes of data. It’s like having the right spice for every dish in your kitchen!
-
How can data scientists balance data security and accessibility?
Balancing data security and accessibility is like doing yoga. It requires balance, flexibility, and a calm mind. Using secure platforms, implementing access controls, and staying updated with security trends can help strike this balance.
Resent Post
>
How to Crack the CPA Exams in Your First Attempt: Proven Tips That Actually Work
>
Top ACCA Course in Chennai with Fees, Course Details & Pros and Cons
>
Top 10 Mistakes Students Make in CFA Level 2 (And How to Avoid Them) Study Plan, Tips & Must-Know Tricks
>
Financial Planning Made Simple: Everything You Need to Know
>
Is a Financial Modeling Course Really Worth It in 2025? Here’s the Real ROI
Follow Us For All Updates!